To give you an idea what each Keyword provider is offering we’ve made a compilation from a variety of photos. In general: MyKeyWorder delivers the most keywords but they have no confidence level, whereas Google and Microsoft are more cautious and more accurate.
We offer 2 editions for Microsoft Vision: a version where only the best keywords are selected (confidence greater or equal than 5) and a version where all keywords are delivered: all keywords start with a confidence prefix: you can decide for yourself which confidence levels you want to use.
To our opinion: “Microsoft Vision all keywords” is the best offer, because you get all keywords (tags) already qualified with a confidence level. Especially if you’re interested in certain colors it can be very helpful (e.g. col_back_White; col_fore_White;)
That’s why we choosed “Microsoft Vision all keywords” as the standard TAGGER.biz package.
The other options can be ordered as ADD-ON.
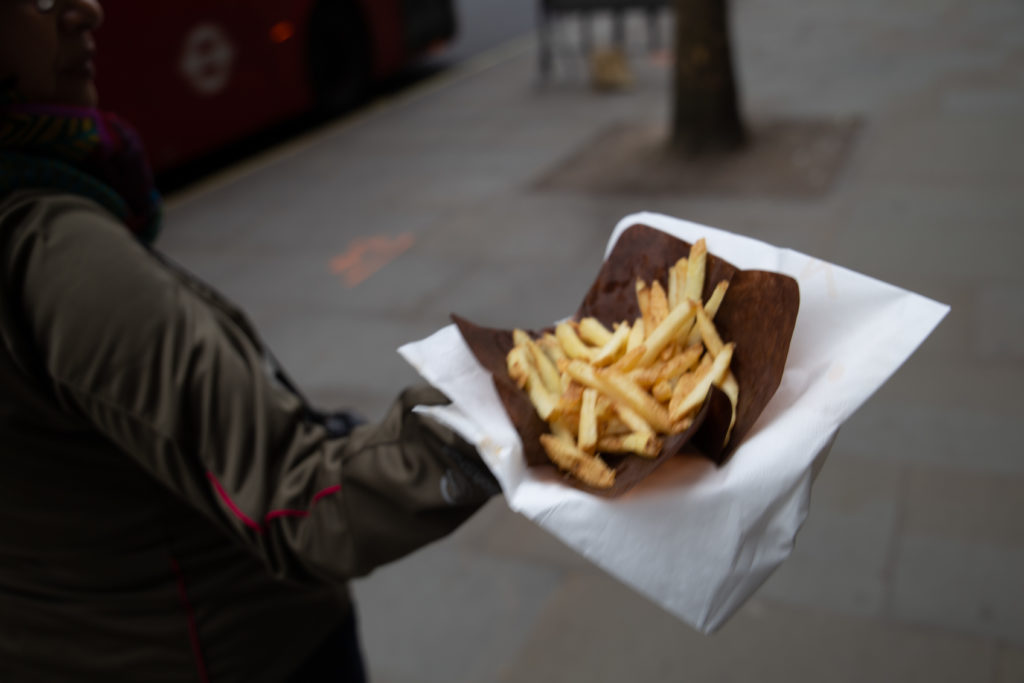
Google Vision
*Cuisine; *Deep_frying; *Dish; *Finger_food; *Fried_food; *Gesture; *Recipe; *fast_food; *food; *tableware; xmp_tagger_biz;
Microsoft Vision Confidence
*French_fries; *Junk_food; *Snack; *fast_food; *food; *sandwich; xmp_tagger_biz;
Microsoft Vision all keywords
0_cheese; 0_coffee; 0_cup; 0_dog; 0_donut; 0_doughnut; 0_food; 0_fries; 0_holding; 0_hot; 0_hotdog; 0_laptop; 0_laying; 0_man; 0_paper; 0_pizza; 0_plate; 0_sandwich; 0_sitting; 0_table; 0_woman; 5_sandwich; 6_junk_food; 9_fast_food; 9_food; 9_french_fries; 9_snack; cat_0_others_; col_Black; col_Grey; col_back_Grey; col_fore_Grey; description_a_sandwich_sitting_on_top_of_a_table; xmp_tagger_biz;
MyKeyWorder
*Beer; *Child; *Dinner; *Fish; *French_fries; *Lunch; *Restaurant; *city; *delicious; *drag_race; *fast; *food; *indoors; *ketchup; *man; *no_person; *people; *street; *unhealthy; *woman; xmp_tagger_biz;
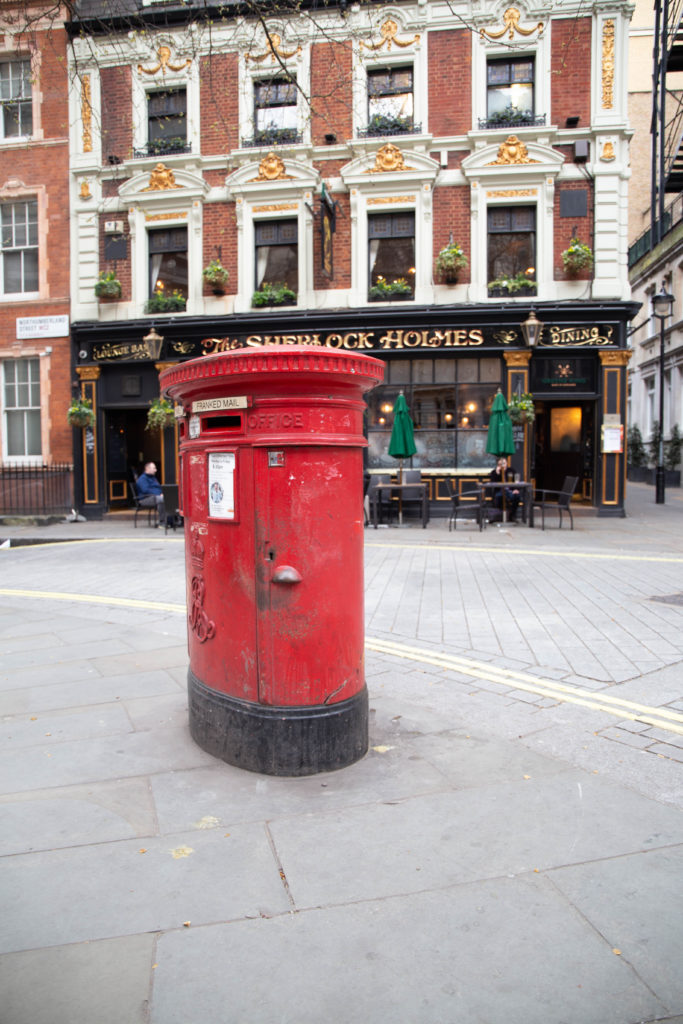
Google Vision
*Asphalt; *Fixture; *Gas; *Mailbox; *Post_box; *Public_space; *Road_surface; *building; *sidewalk; *window; xmp_tagger_biz;
Microsoft Vision Confidence
*Gas; *Mailbox; *Post_box; *building; *city; *fire_hydrant; *ground; *outdoor; *street; *text; *waste_container; xmp_tagger_biz;
Microsoft Vision all keywords
0_brick; 0_building; 0_bus; 0_car; 0_city; 0_driving; 0_green; 0_hydrant; 0_intersection; 0_large; 0_man; 0_old; 0_outdoor; 0_parked; 0_side; 0_sidewalk; 0_sign; 0_sitting; 0_standing; 0_stop; 0_street; 0_tall; 0_track; 0_traffic; 0_train; 0_walking; 5_gas; 5_mailbox; 6_city; 6_post_box; 7_text; 8_fire_hydrant; 8_street; 9_building; 9_ground; 9_outdoor; 9_waste_container; cat_0_outdoor_; cat_1_text_sign; col_Grey; col_White; col_back_White; col_fore_White; description_a_city_street; xmp_tagger_biz;
MyKeyWorder
*Architecture; *Facade; *Tourism; *Town; *building; *city; *door; *exterior; *house; *no_person; *old; *outdoors; *pavement; *road; *street; *traditional; *travel; *urban; *vintage; *window; xmp_tagger_biz;
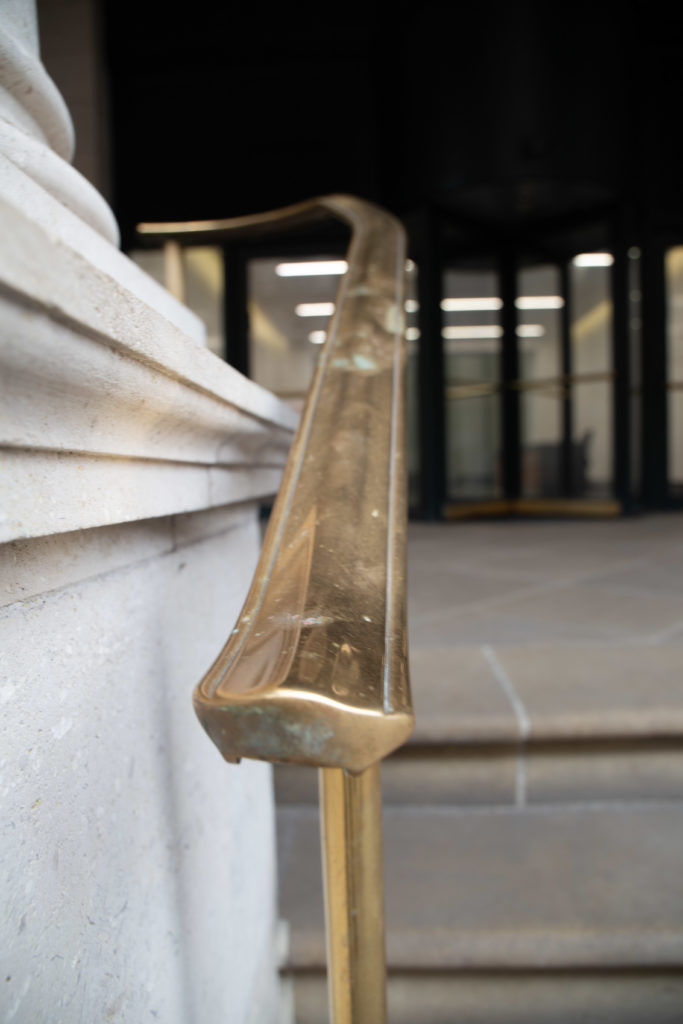
Google Vision
*Composite_material; *Natural_material; *Plywood; *Steel; *Transparency; *glass; *metal; *room; *wood; xmp_tagger_biz;
Microsoft Vision Confidence
*metal; xmp_tagger_biz;
Microsoft Vision all keywords
0_bed; 0_bench; 0_building; 0_cat; 0_chair; 0_holding; 0_laying; 0_man; 0_room; 0_sitting; 0_standing; 0_table; 0_umbrella; 0_wooden; 7_metal; cat_0_abstract_; cat_0_others_; cat_0_outdoor_; col_Black; col_Grey; col_White; col_back_Grey; col_fore_Black; description_a_chair_sitting_in_front_of_a_building; xmp_tagger_biz;
MyKeyWorder
*Architecture; *Facade; *Tourism; *Town; *building; *city; *door; *exterior; *house; *no_person; *old; *outdoors; *pavement; *road; *street; *traditional; *travel; *urban; *vintage; *window; xmp_tagger_biz;
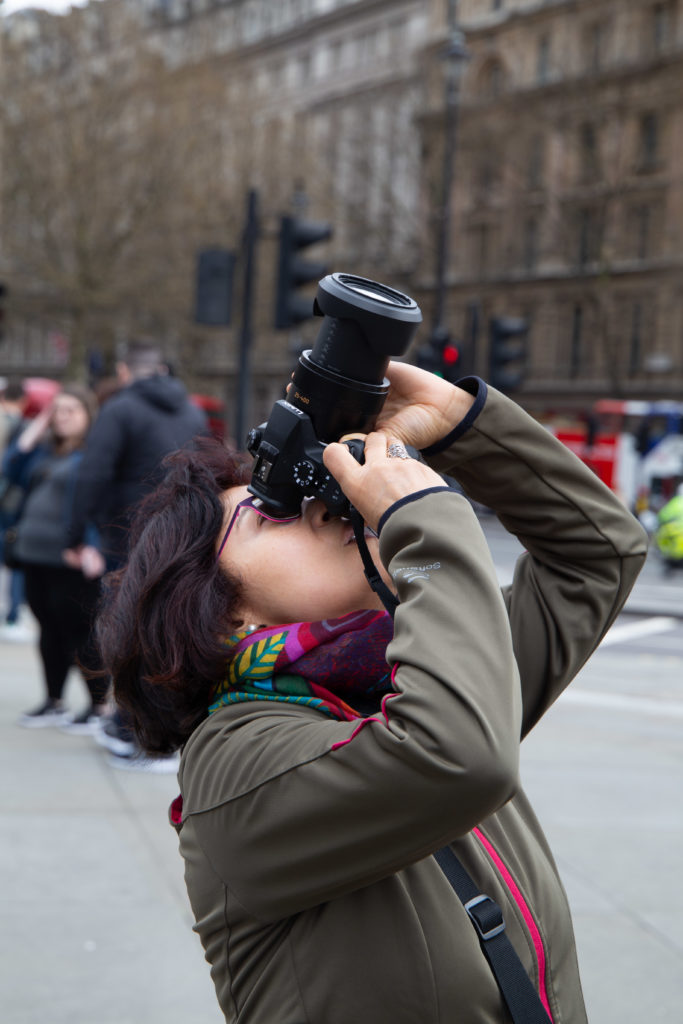
Google Vision
*Camera; *Cameras__and__optics; *Digital_camera; *Photographer; *Reflex_camera; *Single-lens_reflex_camera; *Street_fashion; *jacket; *travel; *tree; xmp_tagger_biz;
Microsoft Vision Confidence
*Camera; *city; *clothing; *crowd; *outdoor; *person; *street; *woman; xmp_tagger_biz;
Microsoft Vision all keywords
0_board; 0_building; 0_cellphone; 0_city; 0_crowd; 0_doing; 0_game; 0_group; 0_holding; 0_man; 0_outdoor; 0_people; 0_person; 0_phone; 0_riding; 0_road; 0_sidewalk; 0_snow; 0_standing; 0_street; 0_walking; 0_wearing; 0_woman; 0_young; 5_woman; 7_street; 8_camera; 8_city; 8_clothing; 9_outdoor; 9_person; cat_0_others_; cat_3_people_crowd; cat_3_people_many; col_Black; col_Grey; col_back_Grey; col_fore_Black; description_a_person_that_is_standing_in_the_street; xmp_tagger_biz;
MyKeyWorder
*Journalist; *Lens; *Military; *Police; *action; *battle; *binoculars; *city; *drag_race; *landscape; *man; *offense; *outdoors; *paparazzi; *people; *portrait; *street; *urban; *winter; *woman; xmp_tagger_biz;
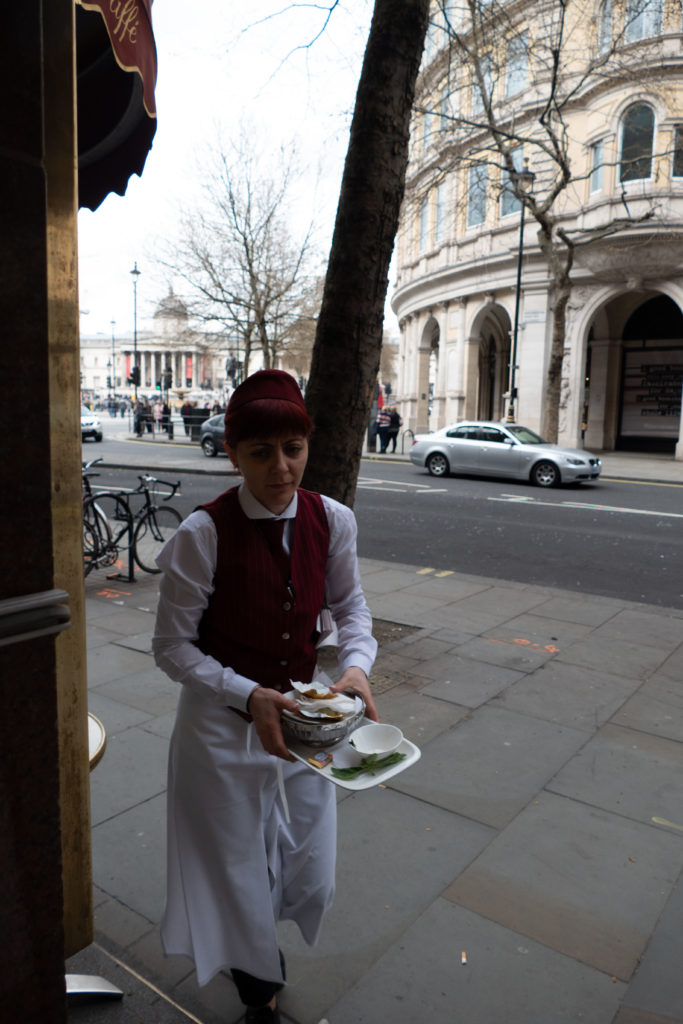
Google Vision
*Asphalt; *Gesture; *Road_surface; *Street_fashion; *building; *car; *sky; *tree; *vehicle; *window; xmp_tagger_biz;
Microsoft Vision Confidence
*car; *clothing; *fast_food; *food; *land_vehicle; *outdoor; *person; *sidewalk; *vehicle; xmp_tagger_biz;
Microsoft Vision all keywords
0_building; 0_city; 0_fire; 0_holding; 0_large; 0_man; 0_outdoor; 0_person; 0_riding; 0_road; 0_sidewalk; 0_standing; 0_street; 0_walking; 0_wearing; 0_woman; 0_young; 6_car; 6_food; 6_sidewalk; 7_fast_food; 7_land_vehicle; 7_vehicle; 8_clothing; 9_outdoor; 9_person; cat_2_outdoor_street; cat_4_building_arch; col_Black; col_Grey; col_White; col_back_Grey; col_fore_White; description_a_man_standing_on_a_sidewalk; xmp_tagger_biz;
MyKeyWorder
*Child; *adult; *city; *daylight; *girl; *lid; *man; *one; *outdoors; *people; *portrait; *religion; *road; *street; *travel; *urban; *veil; *wear; *wedding; *woman; xmp_tagger_biz;
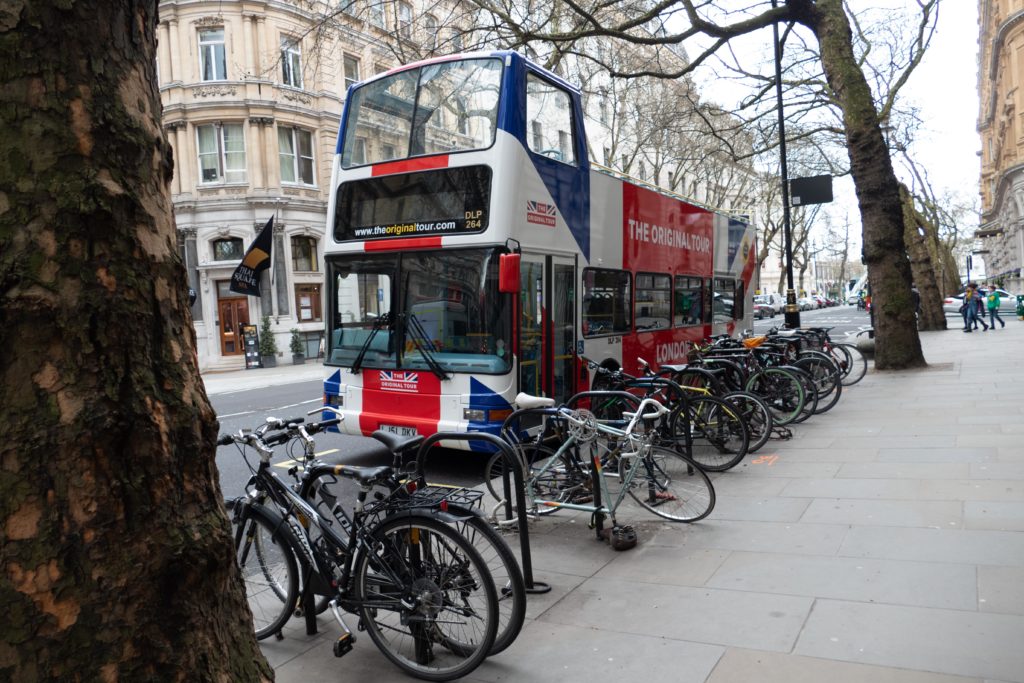
Google Vision
*Bicycles–Equipment_and_supplies; *Bus; *bicycle; *bicycle_tire; *bicycle_wheel; *land_vehicle; *tire; *vehicle; *wheel; *window; xmp_tagger_biz;
Microsoft Vision Confidence
*bicycle; *bicycle_wheel; *bike; *city; *land_vehicle; *outdoor; *sidewalk; *street; *tree; *vehicle; *way; *wheel; xmp_tagger_biz;
Microsoft Vision all keywords
0_bicycle; 0_building; 0_bus; 0_busy; 0_city; 0_decker; 0_double; 0_group; 0_large; 0_man; 0_narrow; 0_outdoor; 0_parked; 0_people; 0_riding; 0_road; 0_sidewalk; 0_small; 0_standing; 0_street; 0_train; 0_walking; 0_woman; 0_young; 2_sidewalk; 4_way; 5_bike; 7_city; 7_street; 8_bicycle_wheel; 8_wheel; 9_bicycle; 9_land_vehicle; 9_outdoor; 9_tree; 9_vehicle; cat_9_trans_bicycle; col_Black; col_Grey; col_White; col_back_Black; col_fore_Grey; description_a_person_riding_a_bicycle_on_a_city_street; xmp_tagger_biz;
MyKeyWorder
*Bus; *Town; *Traffic; *bike; *city; *commuter; *cyclist; *daylight; *no_person; *outdoors; *people; *public; *road; *sightseeing; *street; *transportation_system; *travel; *urban; *vehicle; *wheel; xmp_tagger_biz;
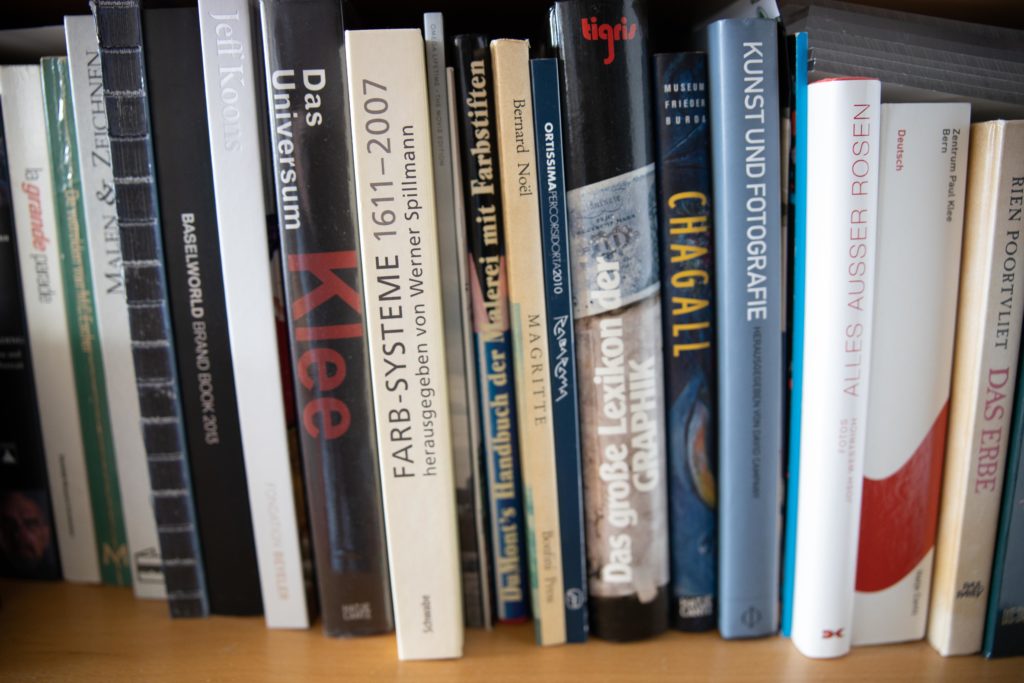
Google Vision
*Collection; *Font; *Material_property; *Shelving; *book; *bookcase; *library; *publication; *shelf; *wood; xmp_tagger_biz;
Google Text
*txt_1149MH__III; *txt_300; *txt_AUSSER_ROSEN; *txt_Das_groe_Lexikon_der_GRAPHIK; *txt_KUNST_UND_FOTOGRAFIE; *txt_Tell_her; *txt_Universum; *txt_sea_FARB_-_SYSTEME_1611-2007_engegeben_von_Werner_Spiliman; xmp_tagger_biz;
Microsoft Vision Confidence
*book; *bookcase; *bookshelf; *computer; *indoor; *lined; *lots; *many; *publication; *row; *several; *shelf; *stack; *text; xmp_tagger_biz;
Microsoft Vision all keywords
0_book; 0_bookshelf; 0_bunch; 0_computer; 0_desk; 0_different; 0_filled; 0_front; 0_full; 0_group; 0_indoor; 0_keyboard; 0_lined; 0_many; 0_row; 0_several; 0_shelf; 0_sitting; 0_stack; 0_wooden; 1_several; 2_bookshelf; 2_stack; 7_lots; 7_many; 7_publication; 8_row; 9_book; 9_bookcase; 9_computer; 9_indoor; 9_lined; 9_shelf; 9_text; cat_8_text_; col_Black; col_Grey; col_White; col_back_Black; col_fore_White; description_a_book_shelf_filled_with_books; xmp_tagger_biz;
MyKeyWorder
*Business; *College; *abundance; *book; *bookcase; *bookstore; *data; *education; *facts; *knowledge; *library; *literature; *no_person; *organization; *reference_book; *research; *school; *shelf; *stacks; *university; xmp_tagger_biz;
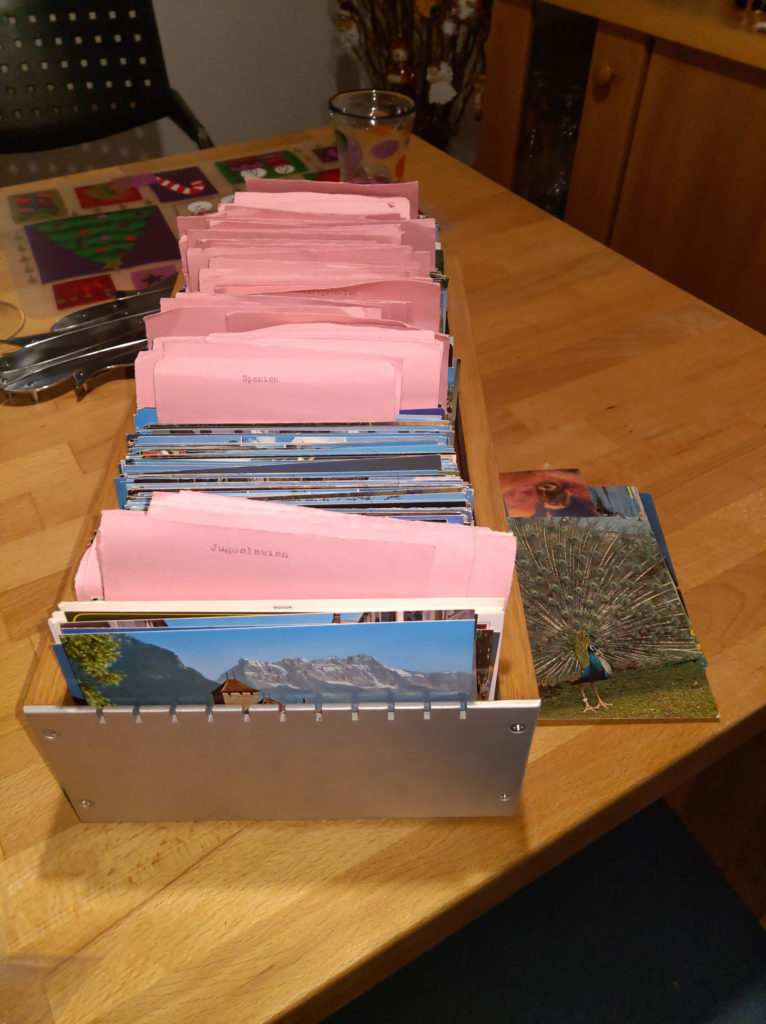
Google Vision
*Flooring; *Hardwood; *Plywood; *Rectangle; *Wood_stain; *cabinetry; *publication; *room; *table; *wood; xmp_tagger_biz;
Microsoft Vision Confidence
*book; *box; *cluttered; *floor; *furniture; *indoor; *open; *paper; *table; *text; *wooden; xmp_tagger_biz;
Microsoft Vision all keywords
0_book; 0_box; 0_computer; 0_cutting; 0_desk; 0_indoor; 0_keyboard; 0_laptop; 0_laying; 0_living; 0_open; 0_room; 0_sitting; 0_small; 0_table; 0_wooden; 1_cluttered; 5_furniture; 5_open; 6_paper; 6_wooden; 8_book; 8_box; 9_floor; 9_indoor; 9_table; 9_text; cat_0_abstract_; cat_0_others_; cat_0_outdoor_; col_Black; col_Brown; col_Pink; col_back_Brown; col_fore_Brown; description_an_open_laptop_computer_sitting_on_top_of_a_wooden_table; xmp_tagger_biz; description_an_open_laptop_computer_sitting_on_top_of_a_wooden_t;
MyKeyWorder
*Business; *Still_life; *book_bindings; *box; *container; *education; *furniture; *indoors; *knowledge; *landscape; *library; *no_person; *organization; *painting; *paper; *people; *school; *university; *wear; *wood; xmp_tagger_biz;
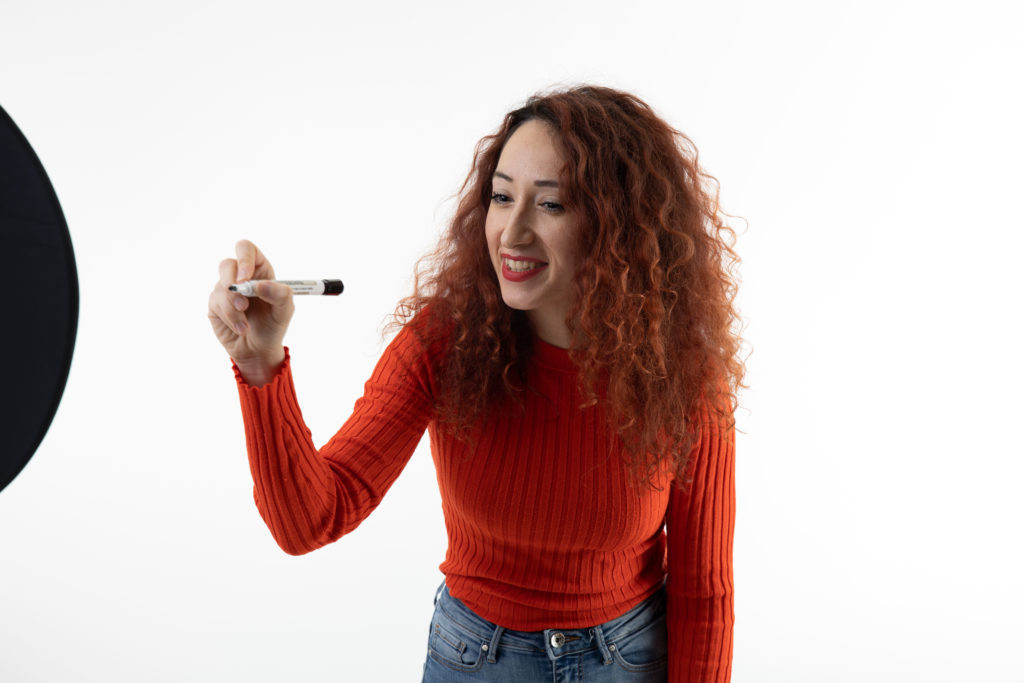
Google Vision
*Elbow; *Flash_photography; *Gesture; *Happy; *Sleeve; *jeans; *lip; *neck; *smile; *waist; xmp_tagger_biz;
Microsoft Vision Confidence
*clothing; *girl; *human_face; *jeans; *person; *smile; *woman; xmp_tagger_biz;
Microsoft Vision all keywords
0_air; 0_cat; 0_doing; 0_hair; 0_holding; 0_indoor; 0_looking; 0_man; 0_mirror; 0_orange; 0_person; 0_playing; 0_room; 0_snow; 0_standing; 0_umbrella; 0_wearing; 0_woman; 0_young; 5_jeans; 6_girl; 6_woman; 8_clothing; 8_human_face; 9_person; 9_smile; Female_26; cat_9_people_; col_White; col_back_White; col_fore_White; description_a_person_standing_in_front_of_a_mirror_posing_for_the_camera; xmp_tagger_biz;
MyKeyWorder
*College; *Music; *Student; *adolescent; *adult; *casual; *contemporary; *cute; *fashion; *friendly; *girl; *hair; *isolated; *joy; *looking; *one; *people; *pretty; *woman; *young; xmp_tagger_biz;
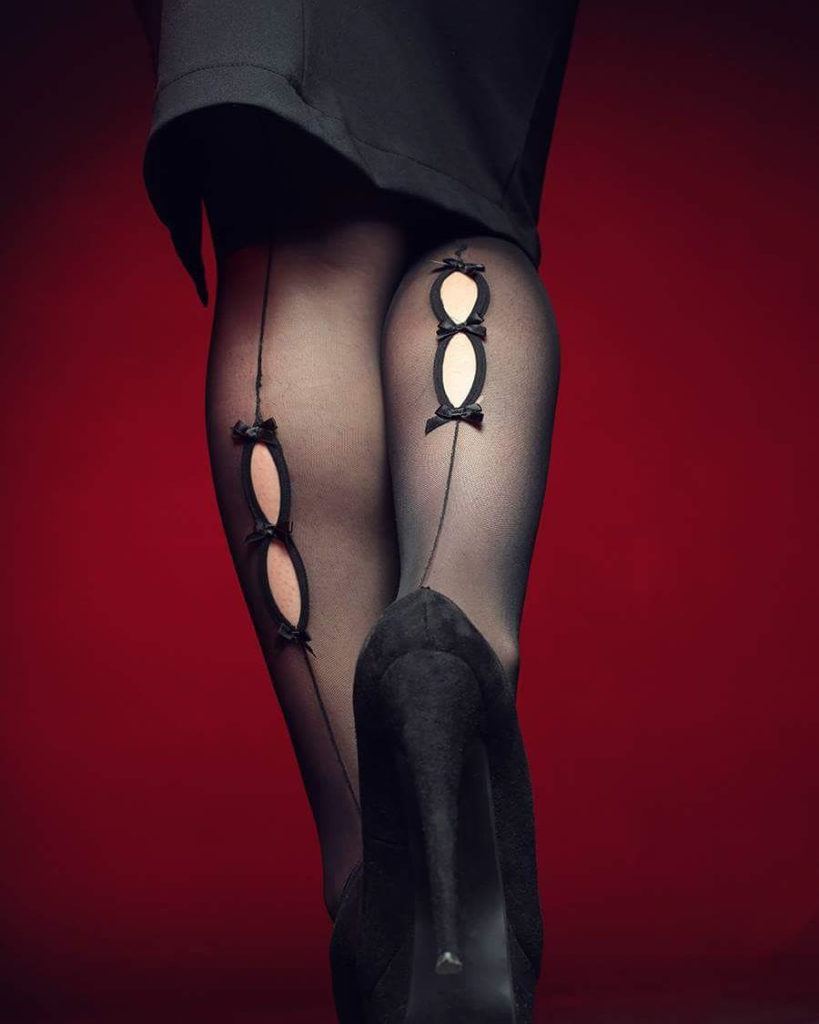
Google Vision
*Calf; *Foot; *Grey; *Human_leg; *Leg; *Shoe; *Sportswear; *Stocking; *knee; *thigh; xmp_tagger_biz;
Microsoft Vision Confidence
*Stomach; *Tattoo; *Undergarment; *art_model; *brassiere; *high_heels; *indoor; *model; *person; xmp_tagger_biz;
Microsoft Vision all keywords
0_blue; 0_board; 0_hat; 0_holding; 0_indoor; 0_man; 0_neck; 0_pair; 0_person; 0_room; 0_standing; 0_suit; 0_table; 0_wearing; 0_woman; 0_young; 5_art_model; 5_model; 5_stomach; 5_undergarment; 6_high_heels; 7_brassiere; 7_tattoo; 8_person; 9_indoor; cat_9_people_; col_Black; col_Red; col_back_Red; col_fore_Grey; description_a_person_wearing_a_costume; xmp_tagger_biz;
MyKeyWorder
*Leather; *Love; *beautiful; *body; *elegant; *erotic; *fashion; *fishnet; *girl; *glamour; *lingerie; *model; *naked; *nude; *pantyhose; *sexy; *studio; *underwear; *woman; *young; xmp_tagger_biz;